Today’s wildly shifting market can make it difficult for marketers to predict changes in customer behavior. It’s easy to say that discounting will attract more impulse purchases for the least amount of risk. But customer behavior modeling offers valuable rational insight into the purchasing decision process that tells us differently.
While discounting may seem like the oldest trick in the book, marketers know that it’s not sustainable. If you advertise 50% off every second week, people will simply learn to wait for the next sale rather than buy at full price. Discounting, when you look at consumer behavior models, ends up canceling itself out.
A better framework focuses on personalization – promoting just the right offers to just the right people, and building an experience that’s the best for them. It’s about curating customer-centric experiences that are based on common behaviors observed through data.
Modeling Customer Behavior
Marketing departments use consumer behavior modeling to get a better understanding of how their customers buy. Considering external factors, impulse buying theory, economic model data, and outcome analysis all help inform better strategic marketing initiatives.
While most marketing experts have come across data modeling before, it’s important to understand how these models can be used to design better personalization for your brand.
Common consumer behavior learning model types include:
- Propensity models
- Affinity models
- Churn models
Incorporating AI into these traditional customer models allows you to gather complete knowledge about particular groups and how they spend money. Better data surrounding external variables means better analysis through these models – and that drives better sales.
Everything You Need To Know About Personalized Customer Engagement
Here’s what you need to know right now about personalization and building better relationships with future customers.
Propensity Models: What Do We Know About Buyer Behavior?
Knowing what products people like means you can create personalized promotions to expand your customer footprint. It also means you can avoid promoting products to loyal customers you know will buy anyway – and instead offer them add-ons or related products that widen their purchase.
The types of data used for propensity modeling are:
- Previous transactions and purchasing behavior
- Propensity to buy similar products within your target audience
- Lookalike audiences and their buying behavior
- Historic purchasing decisions and propensity to buy across different categories
Basic problem solving shows us that if you know Jane loves iced tea, then you can stop giving her discounts on iced tea because you know she already pays full price. Instead, start offering Jane incentives to buy your delicious biscotti for her to enjoy alongside her favorite afternoon drink.
The trick, though, is that you have to know Jane and her preferences. That means you need accurate, all-encompassing customer and contextual data. That’s where extensive problem-solving comes in – and where you need AI technology to gather it effectively into a complex model.
Using a propensity model to define consumer behavior helps marketers better understand existing customers and the current buying process in your store. To take this learning model to the next step, it’s important to break down what purchases occur as impulse buying as opposed to predictable customer behavior.
Using Affinity Models
Affinity models are all about organizational buying behavior and product purchase association. This customer behavior model uses data to predict what items are often bought together or in succession.
For example, an affinity model may suggest that burgers are most often accompanied by the purchase of fries, or that shampoo and conditioner are usually bought at the same time.
Using this model to inform marketing strategies would suggest that discounting shampoo and conditioner at the same time would not be beneficial. Instead, the model suggests discounting just conditioner would influence customers to also buy shampoo.
This psychoanalytical model draws from multiple layers of influencing factors for consumers – including environmental variables, such as where and when a product is being offered. The affinity model taps into the basic needs of customers, the suggested purchase offers that form naturally, and making the most of what consumers tend to do already.
Customer Churn Models: Who’s Likely To Leave, and How Can We Stop Them?
Personalization increases stickiness – and keeping sticky customers costs businesses five times less than acquiring new ones.
Customers who see relevant offers tend to engage further with brands in the following weeks, expecting more personalized offers to follow. To reduce customer churn, a personal variable model focuses on the data about when and why customers leave to begin with.
Churn model data includes:
- Customer activity including purchases and app activity
- When and where customers make pure impulse purchase choices
- Tenure: how long each person has been a customer
As a sociological model, churn analytics unwrap the internal factors that drive the decision-making process for potential customers. Information processing at this scale can be incredibly complex, so it’s important to design your marketing campaign using high-end smart technology.
Engage Your Guest Using Omnichannel Integration and Personalization
Restaurant guests expect a personalized digital experience. Innovating brands must connect with guests to introduce changes that drive both sales and profit.
We’re Already Doing This. How Do We Do It Better?
When you’re only dealing with a few customers, data analysis isn’t a daunting prospect; you can even run your data models on a spreadsheet. But when you have data from 150 million users on your platform, there are better ways to extract a comprehensive model and meaningful insights.
Adding artificial intelligence (AI) and machine learning (ML) to traditional behavior models enables you to predict how similar customers make purchasing decisions and curate personalized buying experiences to keep them engaged.
ML-powered contemporary models learn as they work with data, so as your customers interact with your marketing, your messaging and offers become more targeted and relevant. This type of stimulus response model can also pull in contextual customer data and react to external stimuli a lot quicker than someone manually analyzing a spreadsheet.
Personalized Marketing Works
A consumer behavior model can help tap into superior personalization for your customer, resulting in higher spending, more visits, and fewer churned customers.
When people receive customized offers, they are far more likely to engage with your brand – which helps businesses attract and keep more customers in the long run.
AI- and ML-powered customer behavior models make personalization possible on a massive scale. And since customers are crying out for personalized experiences, the brands that can deliver will come out on top.
Now is the time to take advantage of this growing demand for personalization by investing in the digital tools. Use a customer behavior model to get started, and you’ll begin to see growth across your sales, retention rates, and engagement figures.
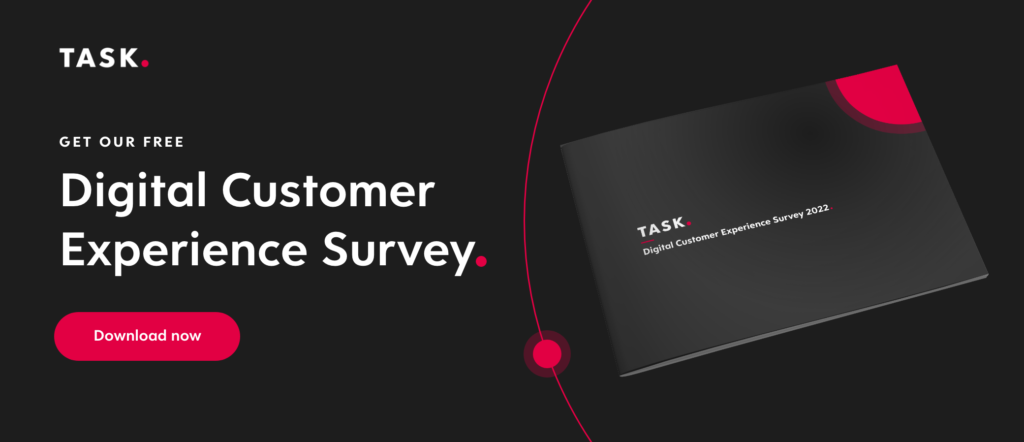